How AI can guess your password
In recent years, Artificial Intelligence has undergone remarkable development, expanding its influence across various domains, including preventive medicine and artistic creations. Cybersecurity is one of the fields where AI-enhanced tools have led to significant improvements and accelerations. A specific example is the automation of processes that were previously performed manually, such as investigations related to Open Source INTelligence (OSINT). OSINT is a practice that focuses on collecting and analyzing information from public and accessible data sources, such as online data, publications, and social media. The goal of OSINT is to gain a comprehensive understanding of specific subjects, organizations, or events by collecting publicly available data, avoiding the use of secret or confidential sources. OSINT is widely used in various sectors, including cybersecurity, digital investigations, intelligence, and risk assessment.
This phenomenon has been influenced by the fact that over time, individuals have increasingly shifted their lives online, dedicating a significant portion of their time to interacting with others through various platforms. This growing online activity has led people to freely share a greater amount of personal information, necessitating a conscious management of such data. For law enforcement, this situation represents an additional source of valuable digital evidence for digital forensic investigations.
Through the use of AI-driven automated OSINT, it has been possible to enhance password cracking techniques. Information obtained from social media research allows for making educated guesses about the passwords of personal accounts. Typically, individuals pay little attention to creating passwords, opting for personal elements such a names, dates, and meaningful objects to facilitate memorization. Leveraging such information significantly reduces the number of passwords to test in order to compromise access to an account. Artificial Intelligence models go beyond extracting information from publicly available online content. Since their primary function in this context is to guess passwords, they are specifically trained to be as efficient as possible in generating realistic passwords.
In this scenario, Generative Adversarial Networks (GANs) come into play. GANs are neural architectures consisting of two artificial intelligence models that operate in mutual competition.
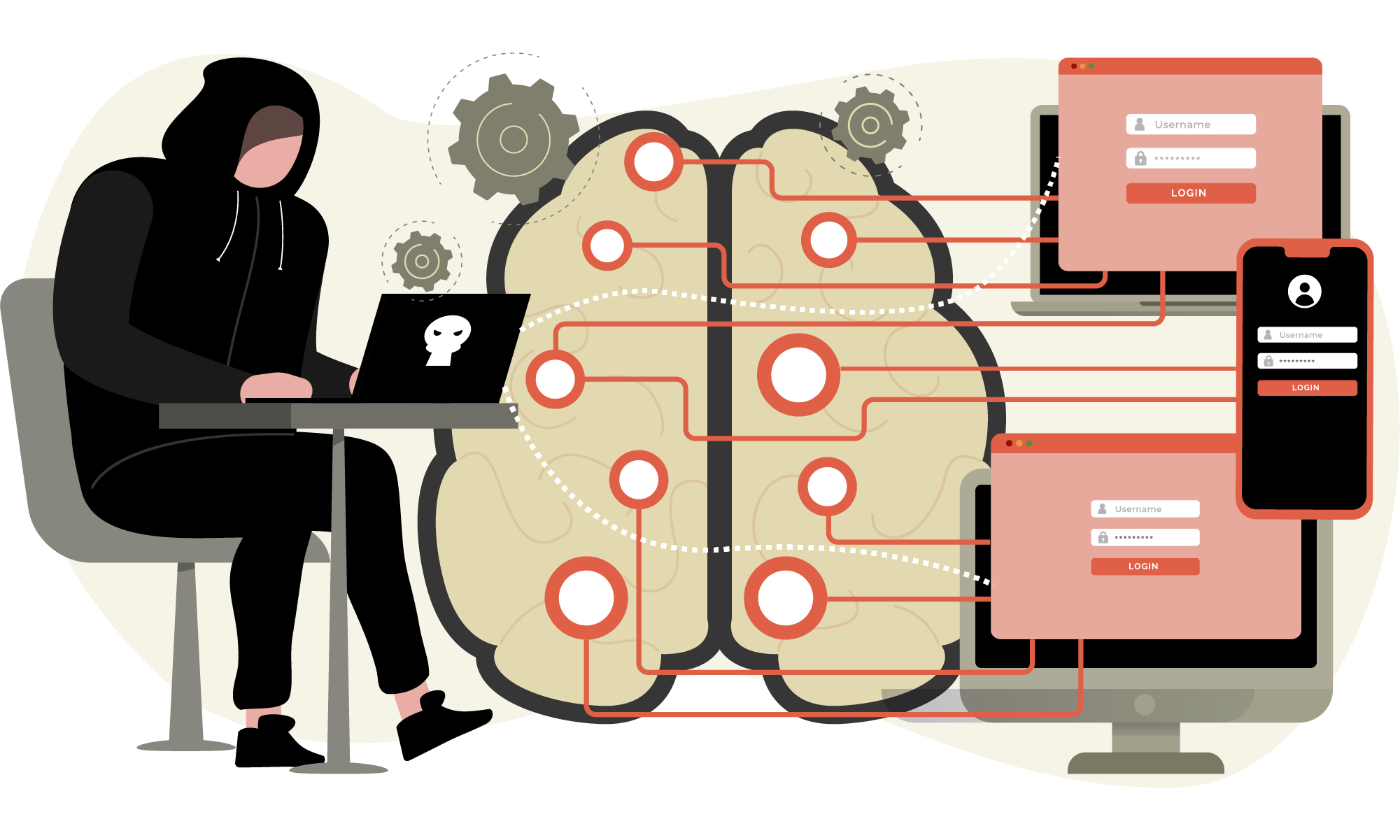
Before proceeding, it’s useful to explain concisely how artificial intelligence works: once the task to be performed is defined, a neural architecture is created to best represent the problem to be solved. Unless working in a research context, one can typically choose the architecture from models already implemented for similar problems. After implementation, the process moves to the training and testing phases, where the new model undergoes learning sessions using databases composed of data similar to what is desired to be learned. These databases already have a defined mapping to help the artificial intelligence understand the purpose of the training. Once the training phase is completed, the testing phase begins, during which the model is fed with unseen data, and its accuracy is evaluated based on the obtained results. Through this testing phase, adjustments to parameters or the architecture itself can be made to get closer to the ideal situation.
Returning to Generative Adversarial Networks (GANs): these are artificial intelligences composed of two architectures with distinct tasks. The discriminator is trained through the training phase using data that provides a foundation to understand the nature of the problem (for instance, by showing images of cats to teach it to distinguish what is a cat and what is not). Subsequently, the second architecture comes into play, the generator, whose task is to deceive the discriminator into believing that the generated data is real and originates from human sources (continuing with the cat example, the generator creates increasingly detailed images in response to the discriminator’s rejection, eventually producing artificial cats so convincing that they deceive the discriminator into believing they are authentic). Upon completion of this procedure, a generator is obtained capable of creating images, data, and passwords similar to those generated by humans.
This tactic has been adopted by PassGAN, which leverages Generative Adversarial Networks (GANs) to autonomously learn distributions of real passwords from legitimate sources, aiming to generate high quality passwords for testing in real-world scenarios. Thanks to the significant efficiency of this approach, it is feasible to integrate the results obtained through the OSINT process to enhance PassGAN’s capabilities in creating plausible passwords for targeted cyber attacks against the designated user.
Given the capabilities of artificial intelligence in this context, the most effective practice to create hard to guess passwords involves adopting various precautions.
These include the unpredictable combination of uppercase and lowercase letters, the inclusion of special characters, and the choice to avoid meaningful words. Additionally, regularly changing passwords and using different credentials for each account significantly contribute to enhancing the overall security of personal data. Fortunately, we are still several years away from an era where artificial intelligences could autonomously hack any account quickly. Therefore, there is no need to worry, but it is still advisable to stay vigilant regarding new advancements in research and development related to data security. A secure method to protect your accounts from theft is to adopt a multi-factor authentication (MFA) system. This technology allows the use of different methods to access your account, making it more challenging for artificial intelligence to guess or compromise the password. In practice, even if an AI manages to overcome one security barrier, it would still be unable to access your account without interacting with a physical secondary authentication device, such as your mobile phone.